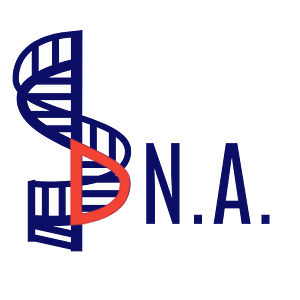
Designing Your Data Narrative
D.N.A
The Designing Your Data Narrative Intensive will provide arts organizations with a compensated opportunity to dive deep into the design and piloting of a data-to-practice process. This component will include 7 online modules and one-on-one sessions with a Data Coach. Data Coaches will be selected by arts organizations from a pool of experts who provide guidance, training, and support in how to effectively work with data.
Designing Your Data Narrative has been specifically developed for arts organizations. Arts professionals will emerge with tools to move from being data collectors to data mentors, demonstrating how data can be used to support strategy, decision-making, and innovation.
By Spring 2024, the arts organizations will have fully engaged in the Designing Your Data Narrative Intensive. Each of these arts organizations will take the lead in presenting an Arts Data Demo to their own networks. This showcase of Arts Data Demos will illustrate how each participating arts organization has harnessed insights gleaned from the training and coaching to shape their very own data-driven stories, setting an inspiring example for their sector.
Meet the 2023/24 Data Cohort
Data AMAs
Inspired by the frequently emulated “Ask Me Anything” format, first popularised on Reddit, we brought in experts during the Designing Your Data Narrative Learning Series to answer key questions on Data Collection, Analysis and Sharing. We’re sharing their answers below to ensure these ideas can be accessed by our community.
Data Collection
With Jen Benoit-Bryan and Katherine Ingersoll, SMU Data Arts
When using surveys, are there any key things to keep in mind around policies to have written in the survey?
Clarity and Transparency: Be clear with respondents about why their opinion matters and how their data will be used. This includes informing them about who will have access to the data and what purposes it will be used for.
Confidentiality: If you’re collecting personally identifiable information, inform respondents about the confidentiality of their responses. This could include stating that their responses won’t be used for fundraising or shared with any other organization.
Contact Information: Provide a contact point (like a general info email) where respondents can go if they have questions or concerns about the survey.
Sharing Results: Consider providing a way for respondents to see what you’ve learned from the survey. This could involve providing a link where they can see key highlights of the survey results after a certain period.
Informed Consent: If you plan to share the dataset itself or release full data tables, it’s important to inform respondents about this potential data use or transfer.
How do we find data we don't have?
There are a lot of datasets available from government sources, such as census data. Sharing data across institutions can be helpful for answering similar questions. For example, if multiple organizations within the same city are all collecting some kind of data that could be in conversation with each other, they might not need to all be collecting that data. They could trade insights to avoid duplication of effort.
Before thinking about the big survey you want to write, it is useful to think about what data you already have. Consider your registration form as a potential source of data.
There are many alternatives to surveys for gathering insights, such as qualitative research with new attenders or subscribers. Smaller sample sizes can still provide valuable insights for comparing different groups within a population.
What methodologies are available to us other than surveys?
There are many alternatives to surveys for gathering insights. These include qualitative research methods such as conducting interviews or focus groups with new attendees or subscribers. She also suggested that organizations can use these methods to check their assumptions and improve the quality of data they’re getting.
For example, organizations can conduct a debrief after an event or program to gather feedback and insights. This can be done informally, such as through a conversation, or more formally through a structured interview or focus group.
Organizations can also use existing data sources to inform their research. This could include analyzing operational data, financial data, or data from registration forms.
What are best practices for maintain privacy and securely storing information?
Maintaining privacy and securely storing information during data collection is key. The first step is to identify whether you’re collecting personally identifiable information. If you are, it’s important to create walls of access between that information and the data you’re using or analyzing. This can be done by separating out the identifiable information, saving a version of the dataset that doesn’t have those fields but has a key identifier so it can be reconnected if needed, and then doing all the analysis on the de-identified dataset.
It is recommended that you get rid of the data after some period of time, for example, six months after a study is complete, to prevent potential future breaches. For more sensitive studies, you can use a “blind” approach where nobody has access to the full dataset and multi-party authentication is required to access the data.
Being clear and transparent with survey respondents about how their data will be used and shared is vital, and it is good practice to provide them with a link to see the key highlights of what the organization learned from their survey responses.
If you want to make the data openly available, what tips or language can you provide to make sure those we are collecting data from are aware of this?
Maintaining transparency by informing respondents about how their data will be used and shared is very important if one wants to make the data openly available. This includes providing the actual underlying raw data set, releasing tables of responses, and informing users of potential data use or transfer. By providing respondents with a link to see the key highlights of what the organization learned from their survey responses, participants are given a sense of payoff and understanding of their contribution,
Are there any major biases that you see come up repeatedly in question creation when collecting data?
Leading Questions: These are questions that prompt or encourage the desired answer. For example, “Isn’t it great that x, y, or z?” or “The program you attended was fantastic, right?” These types of questions can influence how respondents answer.
Double-Barreled Questions: These are questions that ask two things at once. For example, “Was your experience meaningful and enjoyable?” When you include two concepts in one question, it’s hard to interpret which one the respondent is answering.
Question Effect Order: The order in which questions or response options are presented can influence how people respond. The first item on a list is a little more likely to be selected than others. To control for this bias, some survey platforms allow you to randomize the order of response options.
Overloading Questions: Sometimes people try to include too many ideas into one question, which makes it hard to interpret the responses. For example, a question might include 15 or 20 different response options for people to read through. In such cases, respondents are likely to find the first option that sort of applies and pick it.
To mitigate these biases, use clear and concise language, avoide double-barreled questions, and constrain response options to 5-7 options.
Is there a particular survey that was really well written that you've seen and would want people to reference?
Check out the survey toolkit from Of/By/For All that helps organizations design surveys for different constituencies.
What are some data collection best practices?
- Understand the target population and sample size needed to be representative. Consider using census data for comparison.
- Separate personally identifiable information from the dataset for analysis to protect privacy. Remove this information after the study.
- Be transparent about how data will be used and shared. Inform respondents about confidentiality, data access, and opportunities to see results.
- Incentivize survey participation through timely responses, gift cards, or experience-based incentives like behind-the-scenes tours.
- Test surveys with colleagues and cognitive interviews to improve question design and catch biases.
- Use clear, concise, and unbiased language. Avoid double-barreled questions and constrain long lists of responses.
- Consider qualitative research methods like interviews alongside surveys to understand reasons behind responses.
How do we balance qualitative and quantitative data collection, analysis, and presentation well?
Qualitative and quantitative data are powerful when used together. Alternate between the two methods, using quantitative data to identify patterns and then qualitative research to understand the “whys” behind those patterns.
Qualitative research is important for understanding program implementation and addressing potential “blind” spots in quantitative data. Open-ended survey questions and testing surveys qualitatively are some ways to incorporate qualitative aspects into quantitative research.
What are some ways that we can encourage people to contribute to a survey?
- Using incentives like gift cards, as this is a recognized way to boost responses.
- Sending reminders, as response rates often increase with each reminder email sent. Katie noted their surveys rarely get responses on the first email.
- Making the survey invitation timely by asking about a recent experience or relationship, while it’s still fresh in people’s minds.
- Providing an interesting incentive experience, as the speaker shared research found a behind-the-scenes tour incentive was more motivating than a gift card.
- Communicating the value and impact of participation upfront, so people understand how their input can help the organization.
- Testing different incentive options to see what motivates your particular participants or audience the most. Incentives that resonate can vary.
Data Analysis
With Daniel Liadsky and Maia Pelletier, Purpose Analytics
When are qualitative and quantitative method most appropriate to use?
Consider the type of analysis and goals – for example, storytelling versus formal research. Look at the available data and questions being asked to help decide. The analytical question and data availability should guide the choice of methodology. Qualitative and quantitative methods are complementary. Qualitative methods like interviews suit storytelling well, while quantitative counting and summarizing can enhance accountability.
How do you distill interview based data collections down to usable data?
Either keep structured notes during interviews to directly capture answers in a table, or record interviews and then using online tools, transcribe them, code transcripts for themes, and compile the coded tags. Note that it takes practice to identify effective tags. A more basic approach of having some open-text questions in a survey and manually highlighting quotes can be used, too. When analyzing qualitative text data like open-ended survey responses, counting words, keywords and phrases mentioned most frequently can help identify patterns and themes without rigorous transcription.
How do we determine the different levels of importance in data gathering? What is pertinent?
Start with existing data and only collect new data that directly relates to a question you’re asking,
Involve others to challenge assumptions and ensure proposed data gathering aligns with analytical goals.
Each data point collected should directly match and help answer a defined analytical question, rather than just collecting data that may be potentially useful. Only collect data if there is a clear reason and promise to make use of it, in line with principles of data stewardship.
It is important to have a clear analytical plan and research question to guide pertinent data gathering. Test data collection methods with a small group to ensure the data being collected will actually be useful and interpreted as intended.
How do we account for incomplete or small data sets? I.E. we (Yukon Arts Centre) have a very small population of 43,000. Stats Canada results are skewed for us.
Estimating what data is missing is important to understanding the extent of the problem. Start analysis with what is available and gradually add new questions over time. For small datasets, unlock value by finding peers for comparison. Use the Arts Data Platform to compare similar organizations by factors like location, size or discipline to supplement a small internal dataset.
How can AI assist with data analysis? What tools are out there to make data analysis easier?
Online tools that use AI to transcribe audio interviews can help make qualitative coding and theme identification easier. Some survey tools now use AI to help with tasks like keyword extraction from open-text responses, too. However, AI tools still require human review, analysis and interpretation of results, and AI can enhance existing processes rather than replace human analysis.
Do you have any tips or tricks to avoid analyzing data with any preconceived notions?
Keeping data as anonymous as possible by aggregating up from individual levels to avoid seeing names or identifiable details.
Involving others in creating analytical questions to get input and challenges that could address any implicit biases in the questions.
“Gut checking” analysis results against subject matter expertise to evaluate if findings make sense or need further exploration. This helps address any preconceptions influencing interpretation.
Exploring data openly without expectations of specific outcomes, allowing patterns to emerge from the data rather than looking only for expected patterns.
Are there any other ethical considerations you think it’s important to consider?
Data privacy and ensuring individual responses or small groups cannot be identified when sharing analysis results.
Having a plan for how long raw qualitative data like interviews will be stored, as it may contain personal information.
Obtaining clear consent from participants when recording interviews or collecting personal information.
Being transparent about how the data will be used when collecting information from people.
Considering responsible definitions for collected data like gender or racial identity, such as using established sources over creating new definitions.
Data Sharing
With Erica Mattson and Charles Perin
Where do we start? Do we use charts, maps or just lists of numbers?
Start with whatever tools you have available and work to understand your data and resources before deciding how to present it. Identify key data points to convey your most important message before providing deeper layers of more complex data for those interested.
In what ways can data visualizations be made more interactive and engaging for users?
Using sliders to show changes in data over time, allowing viewers to see how metrics change in different time periods
Creating interactive interfaces that allow viewers to explore data on their own through filters and selections, building trust and engagement through self-guided investigation
Leveraging technologies like touchscreens, large displays, virtual reality etc. to create interactive experiences beyond static web or print visualizations
How do you find a balance between simplicity and complexity in visualizations?
Find a balance between simplicity and complexity in visualizations. Trying to include all data and complexity in a single visualization is generally not a good strategy and will overwhelm viewers.
Have you had any experience creating data visuals for funders + government? What might be some things worth considering for this type of audience?
Tailor messaging to understand their specific concerns and interests rather than what arts organizations want to promote
Use jurisdictional comparisons and per capita data to demonstrate need and impact
Show voter support for funding increases through public opinion polling data
Visualize clearly how funding will be spent to demonstrate credibility and accountability
Any tips on how to make data visualizations useful?
Visualizations are useful for exploring uncertainties and generating new hypotheses about relationships in the data, not for answering very precisely defined questions.
They allow interactive exploration of data to help understand it in a way static reports do not.
Visualizations are good for communication if the designer understands the audience and message, but strong conclusions should not be drawn from them alone.
Avoid visualizations that oversimplify the data or are not well-designed as they may backfire and lose the audience’s trust.
Are there tools that assist in colour blind or assisted reading tools for data visualization when showcasing it to an audience?
- Using the GIMP image editor’s color blindness filters to simulate how a visualization would appear to someone with different types of color deficiencies.
- Choosing color schemes and palettes from tools like ColorBrewer that are designed to be colorblind-friendly and distinguishable for different types of data.
- Tableau and other tools will suggest sequential colour ramps that are more accessible than random use of color hues.
What tools are available for data visualization? Are there any online free tools?
Tableau Public (free version available to students and academics) which Charles recommended as one of the best available tools
Excel (basic functionality but widely available)
GIMP image editor (free and open source) for manipulating and simulating visualizations for accessibility
ColorBrewer and similar online tools (free) for choosing accessible color schemes
Want to find out more?
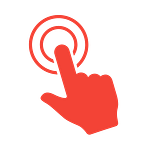
This initiative is made possible through the support of the following



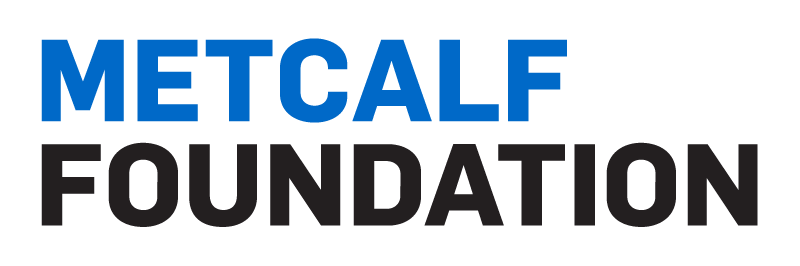
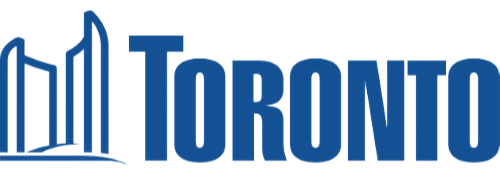
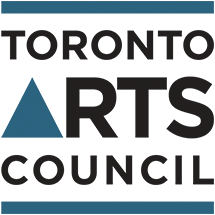
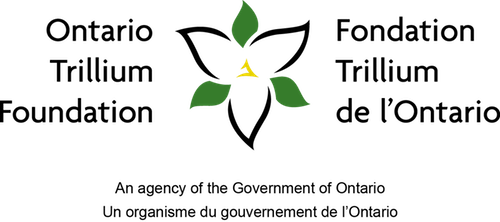
